2 Proven ways to launch successful AI Startups
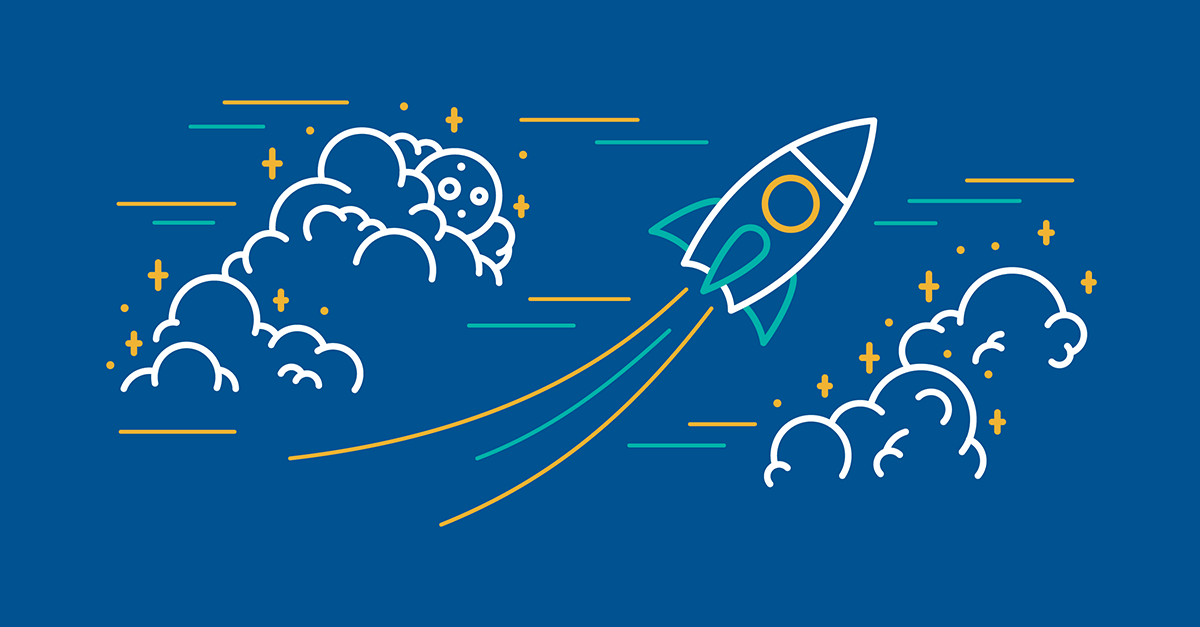
I have seen people struggling to develop AI products because the common methodology to digital products development does not work. It requires some tuning. In this article I will explain two ways to prepare yourself for launching successful B2B AI startups .
To develop a traditional software product, interviews with potential users and preparing clickable wire-frames might be sufficient to scope out a desirable product, after which you can jump into writing the code.
But AI systems require domain understanding, code and data. If you have an idea for, lets say, automating the marketing companions or optimizing capacity planning for a logistics network, you need not only the domain understanding but also marketing campaigns data or logistics data to train a model. This kind of data generally is not available publicly and very fractured in nature. This is why big clouds did not launch the AI solution as a service yet. For example look at AWS, Azure and GCP’s AI managed services such as chatbots, personalizations, forecasting etc.
How do you you get the data that is not easily available and problem domain is relatively small and specialized?
For business-facing (B2B) AI products, it’s often difficult to get the data necessary to build a prototype because a lot of highly specialized data is locked up within the companies that produce it. There are a couple of general ways in which AI teams can get around this problem:
1. Get the Data by providing niche consulting & services.
Some AI teams start by doing NRE (non-recurring engineering services, or consulting) work, in which they build highly customized solutions for a handful of enterprises. You may think this approach doesn’t scale, but you can use it to obtain enough data and domain understanding to learn the lessons or train the models needed to build a repeatable business. Given their need for data, AI startups seem to take this path more often than traditional software startups. I have seen this happening especially in large fragmented industries like healthcare.
2. Get the niche domain understanding by working in vertical markets
Some founders have worked with multiple companies in a vertical market. For example, someone who has worked for a large public cloud company like AWS may have exposure to data and domain from multiple companies in a given industry and witnessed similar issues play out in multiple companies. Imagine an AWS Solutions architect for healthcare, who has worked with tens of healthcare organization for digital and data transformations . This gave him the ability to understand how they operate. what are the problems they are facing and where they investing to create custom solutions?
Experience like this puts founders in a better position to build a nascent product that helps them approach companies that can provide data. Most of the time they already have relationships with their potential client base.
If you lack data to get started on an AI product, these tactics can help you get an initial dataset and domain understanding. Do it yourself or include a co founder in your team who has done one of the above. Once you’ve built a product, it becomes easier to find customers, get access to even more data, and scale up from there.
For consumer-facing (B2C) products, it is generally easier to ask a small group of alpha testers to try out the product and provide data. This may be sufficient to bootstrap the development process. If the data you need is generic to many users, for example, photos on smartphones, spam messages — it’s also likely that any team will be able to find or acquire enough data to get started. But you should not that most of the time Its not going to be a competitive advantage unless you have really large amount of data that no other enterprise can get hold of easily, think the data that Google, Microsoft or amazon has. This this kind of startups are rarely successful.
Member discussion