How to measure and communicate the ROI of data initiatives in your company?
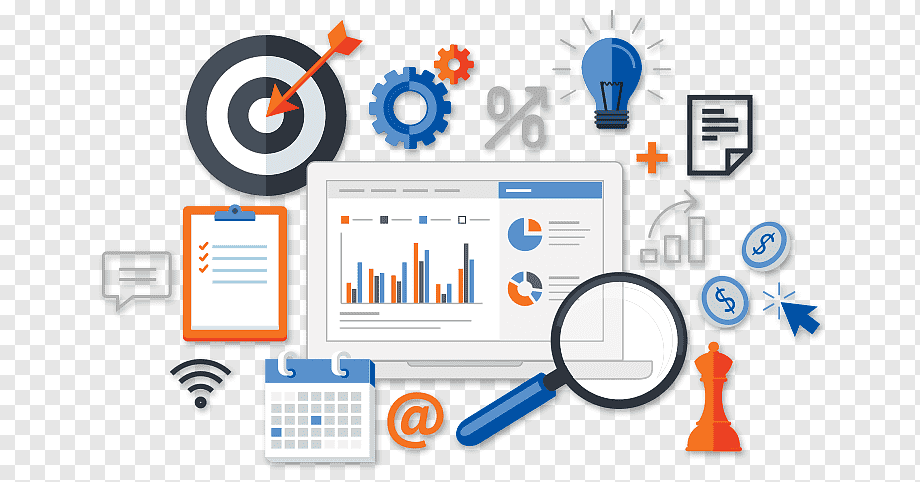
Most data projects do not get realized and sponsored due to ambiguity on how they will help the business. I have seen Data teams far removed from business, due to that they do not understand what value they are generating. I am sharing some ideas on how data project value could be measured and communicated to businesses.
Data Value dashboard
I have seen many successful data leaders develop a value dashboard that compiles and track all ROI information and KPIs that are being impacted by data projects. The main idea is to try to translate the data work they do into dollars and time saved.
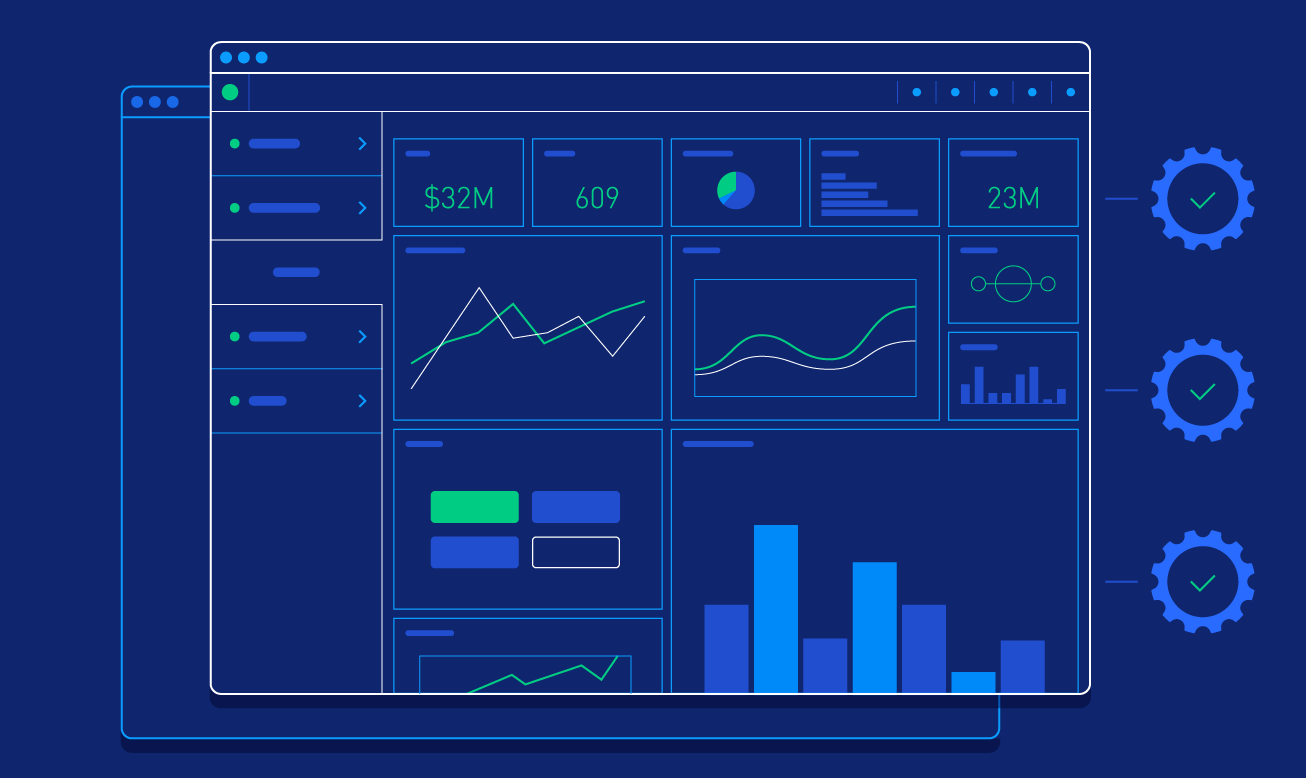
Following are some of the Important metrics to measure data engineering and platforms that can be used for the data value dashboard
Business impact/ROI - This is the most important metric that directly relates to the business value that any data project is generating. All data projects should show some impact in any of the following to be considered successful.
- Has it reduced the cost? - Reduced infrastructure cost, FTE cost, etc, reduced risk of penalties due to non-compliance, improved efficiencies, etc.
- Has it Increased the revenue? - Increased sales, Increased customer retention, reduce customer churn, Improved customer experience, increased customer lifetime value
- Has it improved the business and Technical Agility: Reduced time to experiment with new ideas and new projects, reduced time to ship data projects, reduced efforts to ship a new AI model with a feature store, etc
Some of the technical and operational KPIs are:
Engagement - how often a table/report/model gets queries-baseline it against previous metrics.
Availability- do you find yourself having to shut down the server and restart pipelines? How often were the datasets available at x time every day - define an SLA
Data Quality - Data support queries are increasing or decreasing over time? How many unit tests/data quality checks are going off every day? This indicates the quality of the data
Data Freshness - This is an important metric for internal clients who are looking to consume reports with refreshed datasets. It can be tracked as a part of quality as well. What is the amount of time a critical pipeline is past due for an expected delivery time? Freshness should be tracked at multiple levels, like the quarterly average for critical workload, individual pipeline level, and so on.
Accessibility - How siloes are being removed? what is the amount of queries that are resolved via self-service? how much do cross-teams access the data and also find it useful?
Infrastructure Cost - Is data infrastructure fully optimized? What are the cost per query, cost per user, and cost per TB .....
This is not an exhaustive list, but it will be enough to give you an idea of how to measure data projects.
Member discussion