Why is a data project delivering valuable insights for users still get failed?
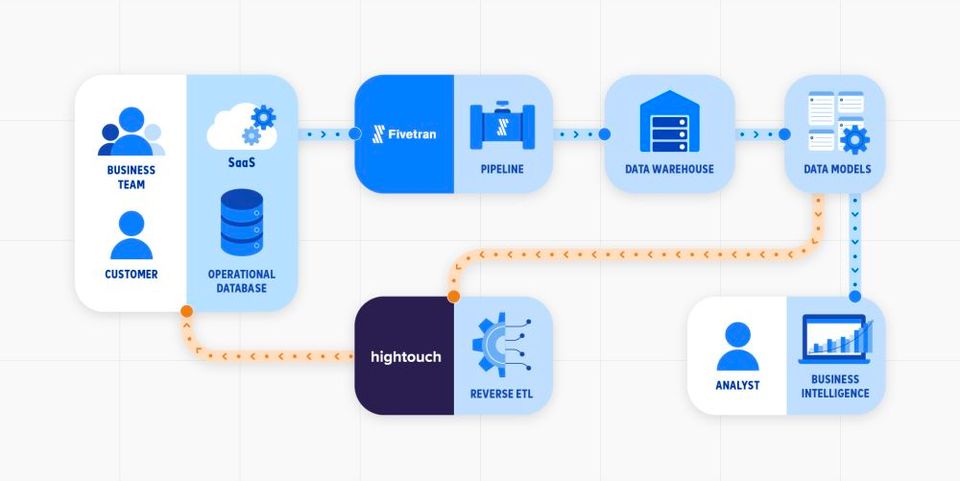
Every company wants to be more data-driven. Yet the most frustrating question for every organization is "how"? Generating insights from data is part that is mostly solved, but the last mile of "analytics enablement" (e.g., translating those insights into action) is being overlooked in many data projects.
The most important output of any data or AI initiative is the insights that are made available for stakeholders, it is kind of like a default, without any insights the initiative would not be called a data initiative.
In my experience, 90% of data projects with good insights still fail, here by failure I mean these initiatives are not get adopted and used by the business users even if the state-of-the-art transformer model is used to predict an insight that is actually valuable for end-users.
Operationalised analytics is about putting an organization’s data to work so everyone can make smart decisions about their business without any friction and significant change in their workflow
This means insights are not made available where end users require them. For example, AI-based Customer segmentation will create friction for users, no matter how useful, if it is not integrated with the CRM and campaign management the end users are using.
There was a valid reason for that, for low-investment AI POCs to be tested for feedback, there was an additional custom API integration work that needed to be done to achieve this, and for many operational legacy systems, the APIs are not available at all.
Even if APIs are available, technology teams have to write their own API connectors from the data warehouse to SaaS products to pipe the data into operational systems like Salesforce, EPIC, SAP, etc. It is hard to write and maintain these connectors because endpoints are brittle and most APIs are not built to handle real-time data transfer. So technology teams need to find way arounds like must set up batching, retries and checkpointing to avoid rate limits. In addition to that mapping fields from the data warehouse to the SaaS product adds another level of complexity and effort.
Why am I saying that problem is solved?
It is solved thanks to increased SaaS tools adoption and emerging reverse ETL tools this is resolved.
In fact, this trend is getting adopted so fast that most modern data architecture designers consider Reverse ETL an essential component of it.
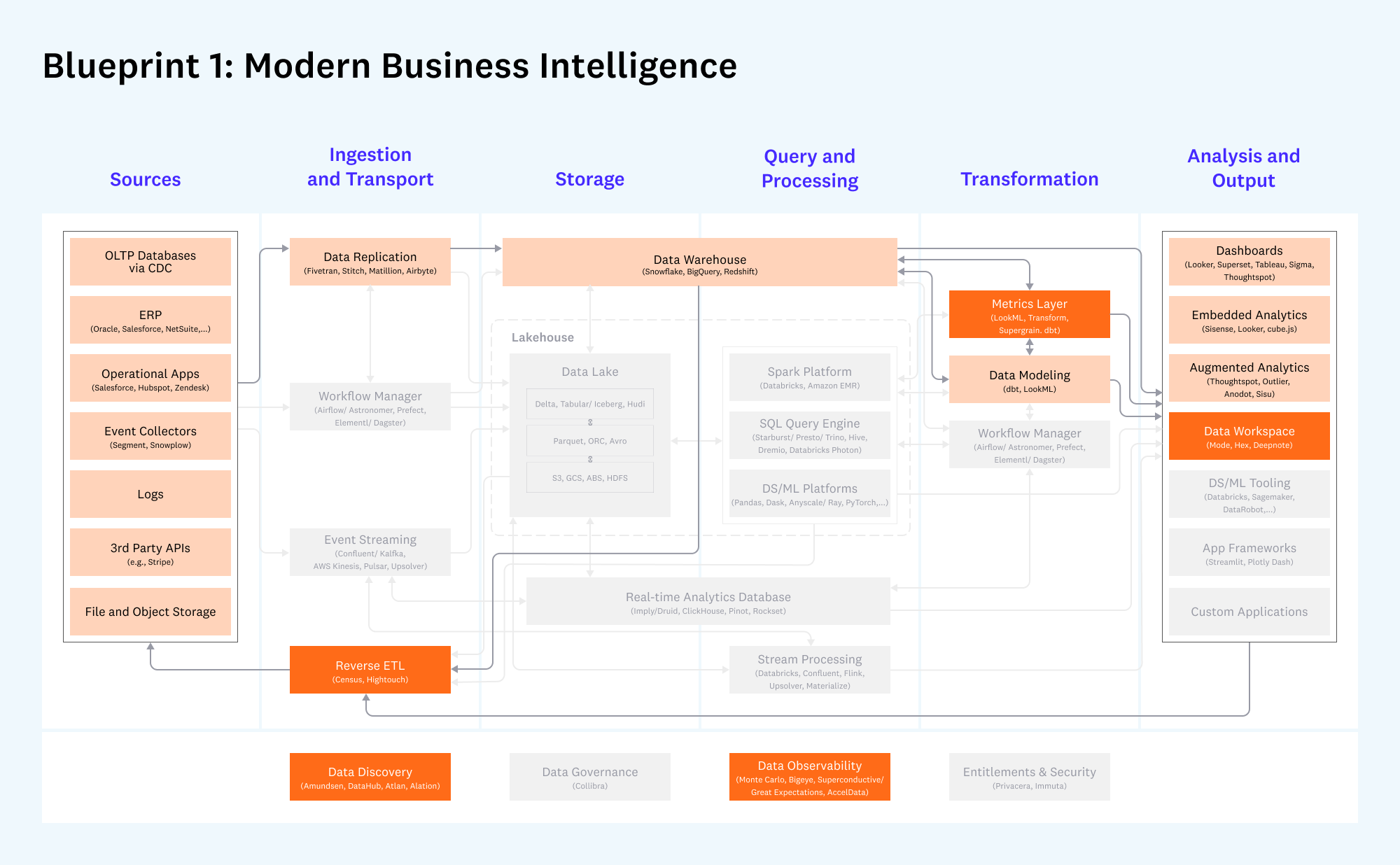
Reverse ETL Tools
The purpose of reverse ETL tools is to update operational systems, like CRM or ERP, with outputs and insights derived from the data warehouse using prebuilt API connectors for most SaaS products.
Without a reverse ETL, the data, and the insights from it, are locked within your BI tools and dashboards. This won’t fly in the era of data-driven companies
Now technology teams do not need to build and maintain custom connectors for the 10s of SaaS platforms in use.
Hightouch and Census are two reverse ETL tools with the widest range of rebuild connectors.
Member discussion